A trusted tool for every modern retailer, the right sales forecast can lead to better purchasing decisions and healthier cash flow. And that’s crucial, because purchasing and cash flow are two of the top 10 areas where most ecommerce businesses fail.
But whether you’re a new or experienced store owner, the task of getting sales forecasting right can leave you feeling overwhelmed — especially when attempting to forecast for new product launches.
With no historical data, it’s tough to know exactly how much inventory you’ll need, and when. Launch with too much product, and you end up with trapped capital and wasted inventory. Launch with not enough, and you risk going out of stock and alienating your new and existing customers.
There’s no way to sugarcoat it, sales forecasting for new products can feel like a catch-22. But luckily, it isn’t.
Today, we’ll reveal how traditional forecasting methods often fall short for new product launches and share the best approach for acing your forecasts, even when you don’t have any past sales data to pull from.
Table of contents
- The problem with forecasting sales for new products
- New product forecasting: How it’s done vs. how it should be done
- Clusterization: A better way to forecast for new product launches
Ready to uplevel your sales forecasting? Get Flieber on your side.
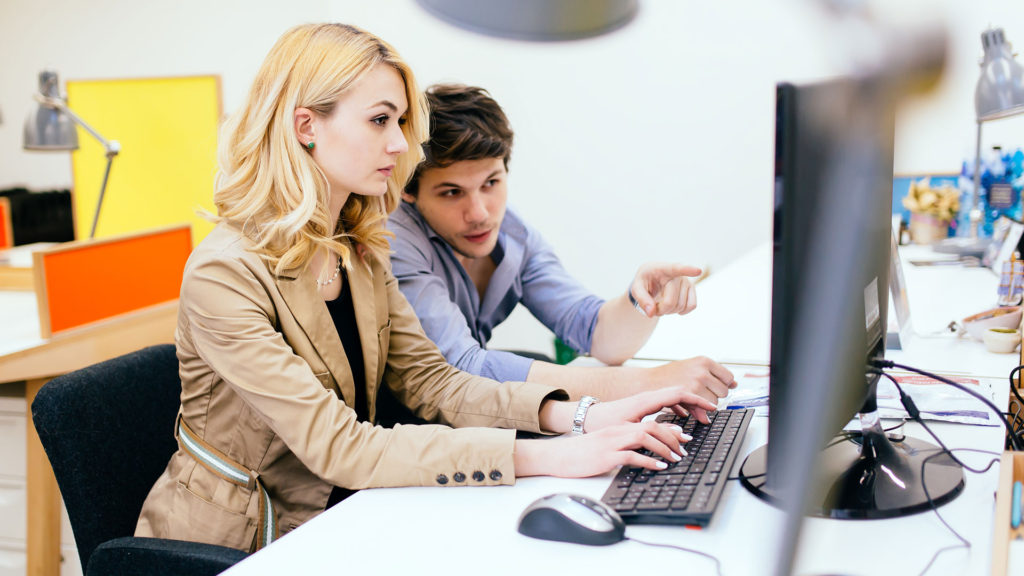
The problem with forecasting sales for new products
At its simplest, a sales forecast predicts how much stock your store will need in the future. But what’s often left out of the discussion is the type of forecast that works best for brand new products with no sales history.
Until recently, online retailers have been forced to find ways of making educated guesses on their stock needs — including tallying up hundreds of competitor product reviews or painstakingly analyzing the sales data for each similar product in their portfolio.
But these methods are extremely time consuming, and they rarely give you an accurate match between projected sales and available inventory. Fortunately, there is a better way.
Sell more products with fewer stockouts. Find out how Flieber can get you back on track
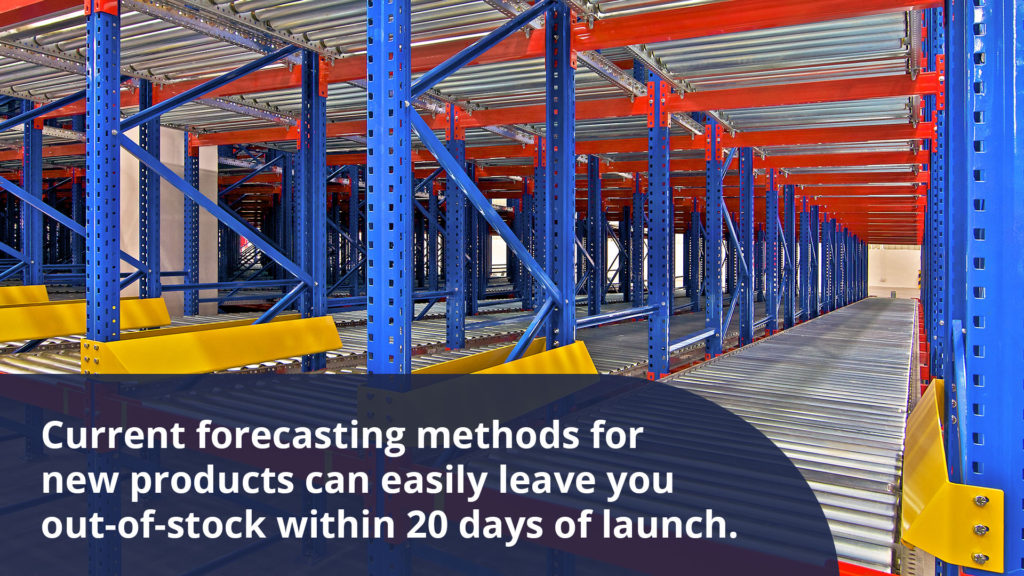
New product forecasting: How it’s done vs. how it should be done
Whether it’s a brand new product or a timeless money maker, the unfortunate truth about forecasting is that 100% accuracy is impossible.
Instead, the secret lies in identifying changes in your sales levels quickly and immediately taking the next best action based on these adjustments.
Let’s take a closer look at the two primary forecasting methods most retailers are currently using when launching a new product to find out exactly where each one falls short.
Method #1 – Sales to Reviews ratio
One of the most common sales forecast practices for new products is scrappy, bootstrappy, and highly manual.
In this scenario, a store owner will physically count the number of customer reviews similar products from their competitors have received in the past month. They’ll then compare that number with their internal sales projections to get a rough ratio of how much stock they’ll need for the launch.
Once you’ve found your Sales to Reviews Ratio, you can then run the opposite math to work out how many units you’ll need.
At face value, it sounds simple enough. But in reality, this method costs you a ton of time and can leave you open to costly mistakes.
Method #2 – The category-based approach
In addition to the Sales to Reviews ratio, many retailers fall back on analyzing an item’s category and subcategories to estimate how much stock they’ll need. And in the case of new products, online retailers tend to over-rely on this method.
That’s because on the surface, categorization looks like a logical approach. After all, if items are in the same category, they should have similar sales, right?
Not exactly. Here’s why:
- Products can fall into the same category but have completely different selling behaviors.
For example, say you own a clothing store and you want to launch a white shirt for men. You base your stock order on the two other shirts in your shop that sell well, which are black and navy. The problem is, if your customers prefer darker colors, your white shirts could end up underperforming. The moral of the story? Categorization can be misleading and cause you to over-order products that won’t sell.
- Category-based forecasts are time-consuming, manual and incomplete.
Not only does category-based forecasting zap your time and energy, it also misses out on valuable data that can help you craft more reliable forecasts. What’s more, because this type of forecast is based on static past data, it doesn’t put you in a position to react quickly if your new product sales rocket or plummet.
- Long lead times can increase required stock volume.
With category-based sales forecasting, you don’t have real-time data guiding your decisions. So, if you have a product with a long lead time, you’ll need a much higher buffer. To put this into context, if your lead time is 5 months for the white shirt, you may decide to order 1,000 units based on sales data from other products in the category. But if you sell 40 per day, you’ll go out of stock in just 25 days. On the other hand, if the market shifts as sales slow, you could be left with too much stock on your hands, and for a long time.
- Category-based forecasts can keep you locked into a vicious stockout cycle.
Because category data is static, you can easily fall into the trap of using the same historical patterns (even ones that include costly stockouts 😬) to make future inventory decisions (often resulting in more stockouts!).
While a category-based approach may feel more intuitive, anyone who’s been in the ecommerce game for long can tell you that regardless of category, no two products ever perform exactly the same. For whatever reason, sometimes a white shirt just won’t sell as well as a navy shirt, despite the fact that they’re in the same category. That’s why at Flieber, we’re big believers in using a clusterization approach based on real-time sales behavior. Here’s how that works.
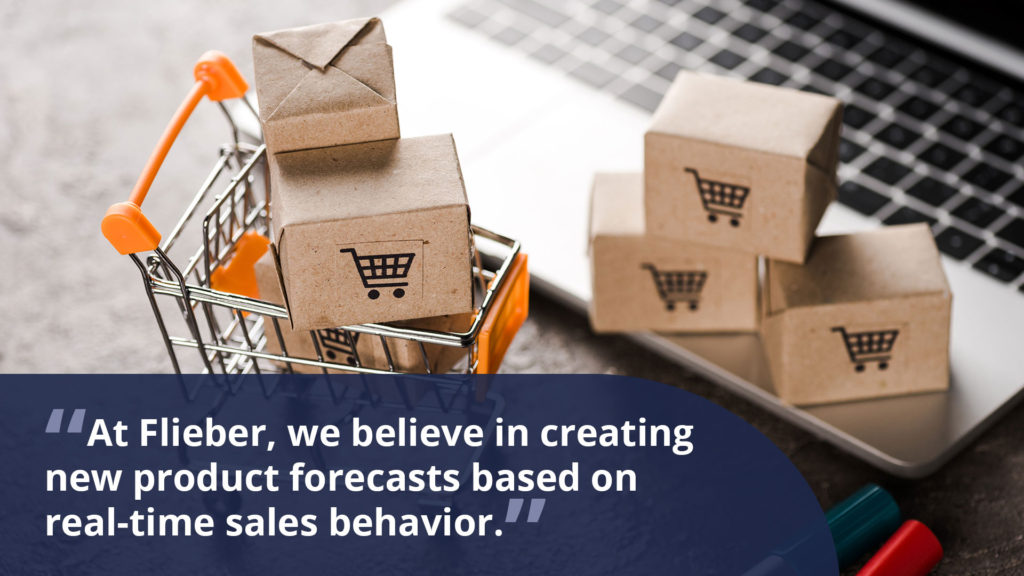
Clusterization: A better way to forecast for new product launches
The clusterization model takes you from a static sales forecasting approach to a more proactive framework that can adapt your inventory needs to your actual sales.
And as a major bonus, there’s a lot less legwork involved.
This modern forecasting approach uses a set of algorithms that groups products by their sales patterns, before putting them into separate data clusters (or ‘buckets’).
Rather than depending solely on category data, clusterization uses patterns in the actual sales behavior of all types of products across a variety of categories to forecast the most likely sales patterns for your new product.
Here’s how the clusterization method works:
Say you have a product that sells 5 units on launch day. Your forecast will identify that this product has a similar sales behavior as pattern A.
On day two, you see that the product has sold 10 units. Now your forecast system will look for patterns where a product sold 2X more units on day two than day one. In this case, let’s say it’s pattern C. Now your system will automatically adjust your forecast from pattern A to pattern C.
Each day, the algorithm analyzes the latest sales data and puts the product in different clusters or ‘buckets’, improving the accuracy of your forecasts until the unit has enough sales data to generate its own highly accurate forecasts.
In machine learning, this is a continuous improvement model known as the ‘next best action’.
Now, the same data framework used by tools like Waze to help you always find the best route based on the most recent information, can help you know exactly how much stock to order for your new product launches. And as a major bonus, the clusterization algorithms get smarter and smarter with each new product you launch.
To recap, a clusterization-based forecast model will:
- Look at existing patterns based on actual sales data.
- Group items with similar sales patterns.
- Create an initial forecast based on the average sales of items in that cluster or ‘bucket’.
- Update the forecast in real time based on the latest sales pattern data from both your new product and similar products in that cluster.
Due to the sheer amount of data to sift through and analyze using clusterization, you’ll need a specialized AI-driven tool to execute this type of forecast. For example, Flieber analyzes and groups over 300,000 products and 20 million sales orders to define the core sales patterns that match the actual behavior of your new product.
Instead of purchasing inventory because you think a product will reach 100 units in the next 30 days, you move to an adaptive framework based on proven sales data.
With clusterization, as soon as you start selling a product, you get alerts to let you know that ‘products like this usually follow this trend’. In most cases, by 10 or 20 days into your launch, you already know whether your new product sales pattern is going up or down and can act accordingly.
New product sales forecasting doesn’t have to be so hard
Category-based is the traditional forecasting approach for new product launches. But that doesn’t mean it’s the right approach.
As online competition increases, maintaining optimal stock levels has never been more important. When you use an optimized sales forecasting method like clusterization, you gain a competitive advantage while reducing costly inventory mistakes and boosting customer loyalty.
Before you know it, manually counting your competitor product reviews will be a thing of the past. With an inventory optimization system that does the heavy lifting for you, you can spend less time worrying about how much inventory you’ll need for your next launch, and more time on generating more revenue for your store.
Ready to make your next launch a success? Find out how Flieber can help.